5 min read
How Data Analytics Is Reshaping Asset Management
Asset management firms are turning big data into opportunities. Here’s how to build a business case for advanced data analytics use cases.
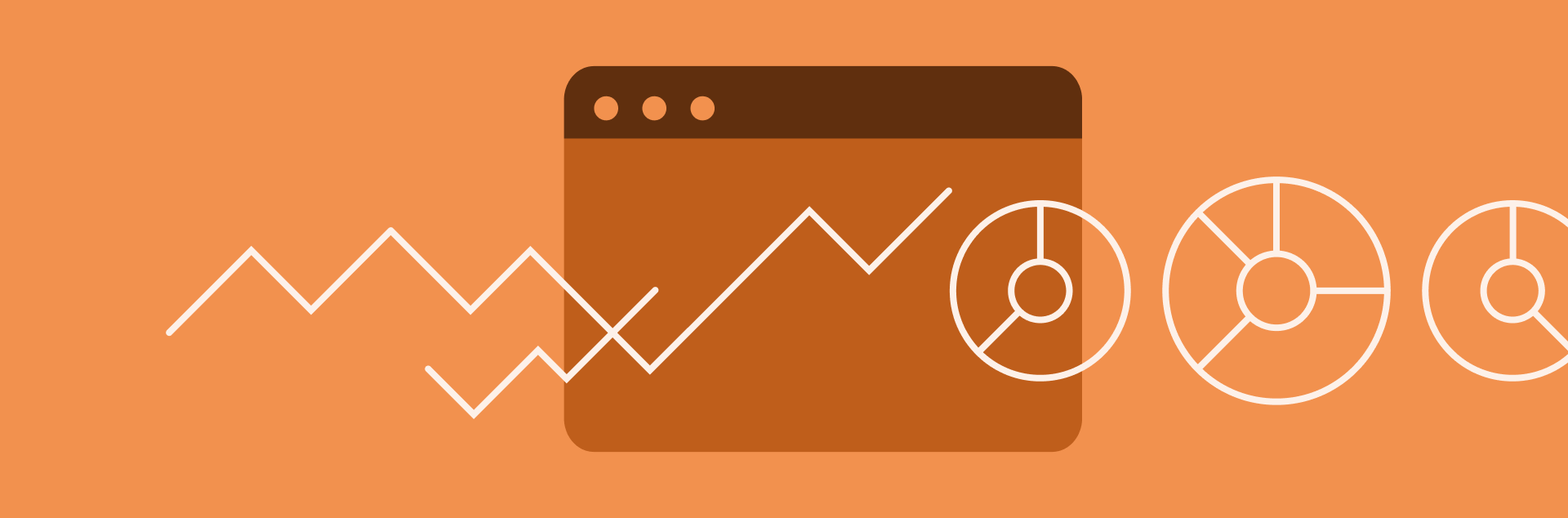
Today’s macro headwinds add pressure when asset managers are already competing in a crowded field.
Market volatility, interest rates, and inflation are making rapid moves unlike anything the industry has seen in a decade. Meanwhile, compressed fees are eating away at margins. Investors are moving money into passive investing and private-market opportunities that not all asset managers can access.
To grow, firms need to find compelling investment opportunities outside the mainstream.
With advanced data analytics, asset managers can survive short-term challenges and build a foundation for long-term growth.
Here’s how asset managers use data analytics to enhance investor outcomes.
How Are Asset Managers Using Data Analytics?
The financial services industry produces petabytes of data a year. It would take over 200,000 iPod Classics to store one petabyte.
With data analytics, asset managers can help sort out signals from static. The right tools could be the difference between gaining a market edge or getting edged out.
Here’s how asset managers are applying data analytics to inform their decision-making.
Market Trend Analysis
Data analytics can decipher market moves, investor behavior, and economic indicators. With advanced tools, analysts can expand their work to consider alternative data points.
These insights are crucial for informed decisions that align with short-term responsiveness and long-term strategic planning. By understanding market movements, managers can capitalize on opportunities as they arise.
Keep up with market trends with Analytics Lab in Morningstar Direct.
Analytics Lab is a flexible coding environment for validating complex investment hypotheses. Using Python, asset managers can innovate with sophisticated statistical analysis and predictive modeling.
Portfolio Management
In one survey, 93% of asset managers said they planned to expand the use of analytics and insights in portfolio management.
Asset managers use data analytics to dissect risk, return, and asset-class correlation. Some apply client data to portfolio optimization, tying products closer to demand.
Descriptive analytics tools help visualize financial metrics to understand portfolio behavior. Asset managers can see and interpret data through visuals like:
- Dashboards with real-time insights into key performance indicators.
- Heat maps that compare performance against peers.
- Correlation matrices that show how investments move together (or don’t).
- Style maps that highlight changes in a portfolio’s profile over time.
- Growth charts that show fund performance based on how $10,000 invested in the fund would have grown over time.
In Morningstar Direct, asset managers can run detailed performance attributions and simulate investment scenarios.
Risk Assessment, Management, and Mitigation
Data analytics helps identify and measure risks to mitigate potential adverse effects.
Firms use data analytics to model stress scenarios and different market conditions. Risk metrics, such as Value at Risk and the Sharpe ratio, can help inform resilient investment strategies.
With the Morningstar Risk Model, users can create custom market scenarios, perform what-if analysis on market movements, and gauge their portfolio exposures to as many as 37 distinct factors.
Trends to Watch in Data Analytics
More than 90% of asset managers are using disruptive technology to enhance investment performance, according to one survey. But they also say this investor expectation is the most challenging to meet.
Leading asset managers have found transformative uses for emerging technologies.
AI and Machine Learning
With artificial intelligence, asset managers can analyze large datasets accurately and efficiently.
Asset managers are using AI and machine learning to:
- Find correlations that could lead to outperformance.
- Forecast asset prices, economic indicators, and market volatility.
- Optimize asset allocation, considering liquidity, transaction costs, and regulatory requirements.
- Assess the impact of market conditions with Monte Carlo simulations.
- Automate rebalancing decisions.
Automation and Real-time Data
In markets where conditions change rapidly, immediate access to data matters.
Automation ensures that data processing is both fast and reliable, reducing the risk of manual errors. Asset managers can make decisions based on the latest data.
Blockchain for Enhanced Data Security
Tokenization of real-world assets is the process of converting traditional assets—such as equities, bonds, real estate, or commodities—into digital tokens on a blockchain platform.
With its decentralized, tamper-proof data architecture, blockchain can secure transaction records. Blockchain could help buy-side asset managers reduce reconciliation errors and improve the data accuracy behind analysis.
Growing Interest in Alternatives
Institutional investors have made private markets a portfolio staple. Now high-net-worth individuals are following suit. In one survey, 62% of respondents said they went outside their primary wealth management relationships to invest in alternatives.
Risk and return analysis that’s routine in the public markets can be challenging in the private markets . Here, companies don’t have to meet the same regulatory disclosure requirements.
Asset managers need to pull data from documents with different formatting. But manual consolidation consumes time and leads to errors.
Data Quality, the Foundation of Effective Analysis
Garbage in, garbage out. Mistyped data entries, data lags, or discrepancies between sources all affect analysis.
Asset managers need to be able to answer these questions about their data:
- Does the data cover a wide range of asset types? Broad coverage supports diversified investment strategies and global market analysis. Our database covers over 800,000 investment offerings across managed investments, equities, abd indexes.
- Is the data correct? Investment data needs to reflect trusted sources, such as regulatory filings. Our data undergoes rigorous validation processes. We uphold data integrity with quality controls ate every stage, from raw data collection to fonal product delivery.
- Is the data provided without unnecessary delay? Asset managers need access to the most current data available. With operations running 24/7, our data factory updates fund prices daily within an hour.
- Is all the data there? Asset managers need complete, dependable insights to lay the groundwork for their decisions. We activate investment offerings only after verifying their comprehensive historical price data.
- Is the data consistent?Firms need data values that appear identical in every instance, regardless of application. We ensure uniformity across all data points to maintain integrity.
Why Data Analytics Initiatives Fail in Asset Management
With narrow margins, analysts need an airtight business case to invest in data analytics. Here’s how to address concerns and avoid all-too-common implementation pitfalls.
"It’s too expensive to invest in data analytics right now."
Evaluate how much prep work the transition requires. Look at all technology options, from flexible APIs that work with current systems to a new platform, to see what adds the most business value. From there, firms can estimate ROI and track progress against goals.
"It’ll take too long to see results."
Flashy pilot projects can fail if their use case seems too narrow. Have leadership set the tone, ensuring initiatives have backing from the top. Start with a project with obvious, broad impact to get the team on board.
"Learning new data analytics skills will be too hard."
Advanced tools are useless if teams don’t know how to use them. The right blend of knowledge requires equity analysts, data scientists, and database managers. Create hands-on training opportunities or make strategic hires to fill gaps.
The Future of Data Analytics in Asset Management
The future of asset management lies in advanced data analytics, backed by high-quality data. As the industry evolves, new technologies will shape effective investment strategies.
Morningstar doesn’t just provide high quality data for asset managers; we also integrate this data into advanced tools to deliver deeper insights into the financial markets.
In the Morningstar Direct investment analysis platform, asset managers can conduct complex portfolio analyses and detailed performance attributions. Simulate potential investment scenarios and strategize accordingly, supported by data-driven insights.
That high-quality data goes beyond the platform. Test complex investment hypotheses in the Analytics Lab sandbox, then bring sophisticated statistical analysis into preferred coding environments with the Python package.
Learn more about how sophisticated data analytics can power your business.